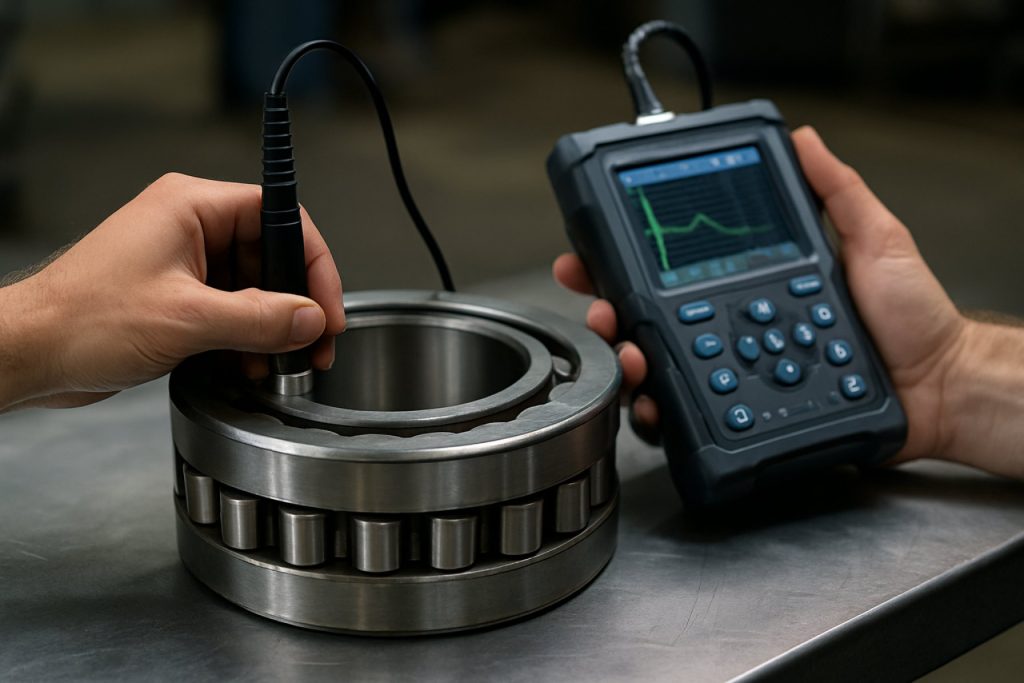
Radial Load Roller Bearing Diagnostics in 2025: How Next-Gen Monitoring Will Slash Downtime, Cut Costs, and Transform the Global Bearings Landscape
- Executive Summary: Key Trends Shaping the Market (2025–2029)
- Market Size, Growth Forecasts, and Revenue Projections
- Emerging Diagnostic Technologies: Sensors, AI, and Real-Time Analytics
- Major Players and Strategic Partnerships
- Industry Applications: Automotive, Industrial Machinery, and Beyond
- Case Studies: Real-World Impact and ROI of Advanced Diagnostics
- Regulatory Landscape and Standards (Referencing asme.org, ieee.org)
- Competitive Analysis: Innovations and Patent Activity
- Challenges and Barriers to Adoption
- Future Outlook: Disruptive Opportunities and Investment Hotspots
- Sources & References
Executive Summary: Key Trends Shaping the Market (2025–2029)
The radial load roller bearing diagnostics sector is undergoing significant transformation as we enter 2025, driven by advances in sensor technology, digitalization, and growing demands for predictive maintenance across diverse industries. Key trends shaping this market from 2025 to 2029 include the integration of Industrial Internet of Things (IIoT)-enabled diagnostic systems, the adoption of machine learning algorithms for early fault detection, and expanding demand from sectors such as wind energy, railways, and heavy manufacturing.
- IIoT-Enabled Real-Time Diagnostics: The proliferation of smart sensors and IIoT platforms is enabling continuous, real-time monitoring of radial load roller bearings. Major manufacturers such as SKF and Schaeffler have been investing heavily in sensor-integrated bearings and cloud-based analytics, allowing users to detect anomalies like lubrication issues, misalignments, and micro-pitting before catastrophic failures occur. This approach supports condition-based maintenance strategies, reducing downtime and maintenance costs for end-users.
- Artificial Intelligence and Predictive Analytics: Sophisticated diagnostic algorithms leveraging AI and machine learning are being increasingly deployed to analyze vibration, temperature, and acoustic data from roller bearings. Companies including NSK Ltd. have introduced solutions that not only flag developing faults but can also estimate remaining useful life (RUL) of bearings, enabling more precise maintenance scheduling and inventory planning.
- Sector-Specific Innovations: The wind energy sector is a key growth market for advanced bearing diagnostics, as turbine reliability is highly dependent on early detection of bearing wear or failure. Timken and JTEKT Corporation are among the suppliers focusing on diagnostic systems tailored for wind turbines and other mission-critical applications, often in collaboration with turbine manufacturers and operators.
- Data-Driven Service Models: The shift toward service-oriented business models—such as remote diagnostics, performance contracts, and lifecycle management—is accelerating. Leading bearing manufacturers now offer digital platforms and remote monitoring centers, providing value-added services that extend beyond hardware supply.
- Regulatory and Sustainability Drivers: Increasing regulatory requirements for asset reliability and a growing emphasis on sustainability are prompting industries to adopt diagnostics that minimize waste and energy use. Early fault detection and predictive maintenance align with these priorities by extending bearing life and improving overall system efficiency.
Looking ahead, the radial load roller bearing diagnostics market is expected to see sustained growth through 2029, as digital tools become increasingly accessible and industries prioritize uptime, safety, and cost efficiency. The ongoing convergence of advanced sensor technology, AI, and cloud computing will continue to redefine best practices in bearing health management.
Market Size, Growth Forecasts, and Revenue Projections
The global market for radial load roller bearing diagnostics is experiencing robust growth as industrial sectors increasingly prioritize predictive maintenance and reliability-centered operations. In 2025, demand for diagnostic solutions is being driven by ongoing digitalization in manufacturing, expansion of the Industrial Internet of Things (IIoT), and the rising need to minimize unplanned downtime in sectors such as automotive, railways, wind energy, and heavy machinery.
Key manufacturers such as Schaeffler AG, SKF Group, and NSK Ltd. are reporting increased adoption of their condition monitoring systems, which leverage vibration analysis, acoustic emission techniques, and smart sensors to provide early detection of bearing defects. These companies are also investing heavily in cloud-based diagnostics and artificial intelligence, anticipating that smart diagnostics will account for a significant share of new installations by 2026.
In 2025, Schaeffler AG, with its Digital Services division, continues to expand its cloud-enabled predictive maintenance platforms, serving industries across Europe, the Americas, and Asia. SKF Group, meanwhile, has rolled out its next-generation vibration sensors and AI-driven analytics, targeting both OEMs and end-users in energy and transport. NSK Ltd. is similarly scaling up its diagnostics portfolio, integrating IoT modules into its bearing systems to enable real-time monitoring and remote diagnostics.
Revenue from radial load roller bearing diagnostics is expected to grow at a compound annual growth rate (CAGR) in the high single digits over the next several years. This outlook is supported by escalating investments in smart factories and the increasing recognition among asset-intensive industries of the cost savings associated with early fault detection. For example, SKF has highlighted that its predictive maintenance offerings can reduce bearing-related downtime by more than 40%, driving ROI for customers (SKF Group).
Asia-Pacific remains the fastest-growing regional market, led by continued industrialization in China and India and growing regulatory pressure for equipment reliability. North America and Europe, meanwhile, are seeing strong replacement demand, particularly in wind energy and rail transport, where operational continuity is critical.
Looking ahead, market participants expect that advancements in sensor miniaturization, edge computing, and machine learning will further accelerate growth, enabling diagnostics to be embedded even in compact machinery and remote assets. As a result, the radial load roller bearing diagnostics sector is positioned for sustained expansion through the remainder of the decade, with established players and new entrants alike investing in R&D and strategic partnerships to capture emerging opportunities.
Emerging Diagnostic Technologies: Sensors, AI, and Real-Time Analytics
In 2025, radial load roller bearing diagnostics are experiencing rapid transformation driven by advances in sensor technology, artificial intelligence (AI), and real-time analytics. The integration of these technologies is enabling predictive maintenance strategies, which are increasingly being adopted across industries such as manufacturing, wind energy, railways, and automotive sectors.
Advanced sensor systems are now at the core of bearing monitoring. High-frequency accelerometers, acoustic emission sensors, and temperature sensors are being embedded directly into bearing housings, enabling continuous data collection under demanding operational conditions. Companies such as Schaeffler Group and SKF have developed smart bearing solutions that incorporate wireless sensor nodes for real-time monitoring of vibration, temperature, and lubrication status. For instance, SKF’s “Insight” technology integrates sensors directly into bearings, facilitating real-time health monitoring and early fault detection, which is particularly useful in critical machinery and remote installations.
AI and machine learning algorithms are increasingly central to extracting actionable insights from the vast data generated by these sensor networks. Deep learning models are now capable of distinguishing between normal operational patterns and early-stage fault signatures in roller bearings, such as spalling, wear, or lubrication breakdown. Timken, a global leader in bearing manufacturing, has expanded its digital portfolio to include AI-powered diagnostic systems designed to predict bearing failures before they result in costly downtime. These systems can analyze both historical and live-streamed sensor data, enabling the implementation of condition-based maintenance schedules.
Cloud-based platforms and edge computing are further amplifying the benefits of these diagnostic tools. Real-time analytics platforms, such as those offered by Schaeffler Group, aggregate data from multiple assets and use AI-driven models to provide fleet-wide health assessments and automated maintenance recommendations. Edge processing, meanwhile, allows for initial data analysis at the sensor or controller level, reducing latency and bandwidth requirements, and ensuring that critical fault detection can occur even in environments with limited connectivity.
Looking ahead to the next several years, the trend is toward even greater sensor miniaturization, increased energy efficiency (including self-powered sensors), and the integration of diagnostics into broader industrial IoT ecosystems. Industry collaborations—such as those between bearing manufacturers and automation technology providers—are expected to accelerate the adoption of fully autonomous, self-diagnosing roller bearing systems. As regulatory and reliability requirements tighten, the demand for robust, real-time, and AI-driven diagnostics in radial load roller bearings is set to grow, shaping maintenance practices and operational safety across sectors.
Major Players and Strategic Partnerships
The landscape of radial load roller bearing diagnostics in 2025 is shaped by the strategic initiatives and collaborations among key industry players, as the sector responds to increasing demands for predictive maintenance and smart manufacturing solutions. Major bearing manufacturers are expanding their diagnostic offerings, leveraging advanced sensor technologies, data analytics, and partnerships with automation and digitalization leaders.
Schaeffler Group, a global leader in bearing manufacturing, continues to invest heavily in digital condition monitoring. Its Optime diagnostic platform integrates wireless sensors for real-time monitoring of radial load roller bearings, supporting predictive maintenance strategies for industrial clients. In recent years, Schaeffler Group has emphasized strategic collaborations with industrial automation firms and has joined consortia for industrial IoT (IIoT) standardization, aiming to enhance the interoperability and scalability of its diagnostic solutions.
Another prominent player, SKF Group, has reinforced its position by integrating AI-driven analytics into its SKF Enlight ecosystem. This platform combines bearing sensors, mobile connectivity, and cloud-based diagnostics to provide actionable insights for radial load roller bearings in sectors such as wind, rail, and heavy industry. SKF Group has announced multiple partnerships with automation giants and digital twin technology providers, aiming to deliver end-to-end asset health solutions. These alliances are expected to accelerate the deployment of remote monitoring and automated diagnostic capabilities over the next few years.
NSK Ltd. is also at the forefront, with its AIP (Asset Improvement Program) offering a comprehensive suite of bearing diagnostics and maintenance planning tools. NSK Ltd. has entered into agreements with major industrial system integrators to embed its sensors and diagnostics into broader factory automation platforms, advancing the adoption of Industry 4.0 standards throughout the sector.
On the supplier side, Timken Company has developed integrated sensor-bearing units and diagnostic software tailored for heavy industrial and rail applications. Recent collaborations with railway equipment manufacturers and process industries signal a focus on asset reliability and digital transformation (Timken Company).
In the coming years, these major players are expected to deepen their strategic partnerships with automation, IIoT, and cloud infrastructure companies, fostering a more connected and intelligent bearing diagnostics ecosystem. This collaborative outlook will likely drive innovations in real-time analytics, remote diagnostics, and lifecycle management, supporting the evolving needs of smart factories and critical infrastructure.
Industry Applications: Automotive, Industrial Machinery, and Beyond
Radial load roller bearing diagnostics play a critical role in ensuring reliability and performance across several key industries, particularly in automotive, industrial machinery, and expanding sectors such as renewable energy and rail. As of 2025, advancements in diagnostic methods—including real-time monitoring, predictive analytics, and sensor integration—are shaping maintenance strategies and operational efficiencies.
In the automotive sector, the increasing complexity of vehicle drivetrains and electrification trends have led to heightened focus on bearing health. Leading manufacturers, such as Schaeffler and NTN Corporation, are investing in embedded sensor technologies that provide continuous data on bearing temperature, vibration, and load. These diagnostics help preempt failures in key components like transmissions and wheel hubs, aligning with the industry’s shift toward predictive maintenance. With the proliferation of electric vehicles (EVs), the load profiles and failure modes of bearings are evolving, necessitating more sophisticated diagnostics and materials.
In industrial machinery, sectors such as manufacturing, mining, and robotics rely heavily on reliable radial load roller bearings in pumps, conveyors, and gearboxes. Companies like SKF Group have launched integrated condition monitoring systems that combine wireless sensors and cloud-based analytics to detect early signs of bearing wear or misalignment. These systems enable plant operators to schedule maintenance during planned downtimes, reducing costly unplanned outages. The use of AI-driven diagnostics is expected to increase, enabling detection of subtle patterns in vibration and acoustic data that may precede more obvious symptoms of bearing failure.
- Railway and Wind Energy Applications: In railways, diagnostics ensure safety and reliability of high-speed trains by monitoring axle and traction motor bearings. Meanwhile, wind turbine manufacturers such as The Timken Company are advancing remote diagnostics for main shaft and gearbox bearings, critical for minimizing downtime in offshore and remote wind farms.
- Trends and Outlook: Across these industries, there is a clear trend toward digitization and integration of bearing diagnostics with broader asset management platforms. Standardization efforts by bodies like ISO and ANSI are supporting interoperability and data exchange, allowing for more seamless diagnostics and maintenance workflows.
Looking ahead, the next few years will likely see increased deployment of edge computing, machine learning, and multi-sensor fusion in radial load roller bearing diagnostics. This evolution will enable more granular, real-time insights, facilitating the transition from reactive to predictive and even prescriptive maintenance models across automotive, industrial, and emerging sectors.
Case Studies: Real-World Impact and ROI of Advanced Diagnostics
The deployment of advanced diagnostics for radial load roller bearings has demonstrated significant real-world impact across various industrial applications in 2025. Case studies from leading sectors such as rail, wind energy, and heavy manufacturing illustrate tangible improvements in operational reliability, maintenance efficiency, and return on investment (ROI).
For instance, Schaeffler AG, a global leader in bearing manufacturing and digital predictive maintenance, has partnered with rail operators to implement condition monitoring systems utilizing AI-driven analysis of vibration and acoustic data from radial roller bearings. In one European rail fleet, the adoption of Schaeffler’s OPTIME system resulted in a documented reduction of unexpected bearing failures by over 40% within the first year, significantly reducing downtime and associated costs. Operators reported a 20% decrease in maintenance labor hours, with ROI realized within 18 months due to the avoidance of catastrophic failures and optimized maintenance scheduling.
In the wind energy sector, SKF Group has collaborated with major wind turbine OEMs to deploy remote monitoring solutions for main shaft and generator bearings, which are often subjected to high radial loads and fluctuating operational conditions. By leveraging real-time data analytics, SKF’s Enlight AI platform enabled early detection of lubrication and alignment issues, extending bearing service intervals by up to 25%. A multinational wind farm owner documented savings exceeding $1.5 million over two years through reduced unplanned outages and extended asset lifespan. The case also highlighted a 30% reduction in emergency maintenance interventions.
Heavy industry has also benefited, as demonstrated by The Timken Company, which outfitted steel mill roller lines with sensor-equipped bearings and cloud-based diagnostics. The deployment enabled maintenance teams to transition from time-based to condition-based maintenance, minimizing unnecessary bearing replacements and production stoppages. Timken’s clients reported up to a 15% increase in bearing life and recouped monitoring investment costs within one annual maintenance cycle due to fewer line interruptions and optimized spare parts inventory.
Looking forward, the integration of machine learning and Industrial Internet of Things (IIoT) is expected to drive further gains. Early adopters anticipate broader adoption of self-diagnosing bearing systems capable of prescriptive maintenance recommendations, further enhancing ROI and operational safety. As manufacturers like Schaeffler, SKF, and Timken expand their digital diagnostics offerings, industry-wide benchmarks for predictive performance and maintenance efficiency are poised to become standard across sectors by the late 2020s.
Regulatory Landscape and Standards (Referencing asme.org, ieee.org)
The regulatory landscape and standards for radial load roller bearing diagnostics are evolving in response to increased industrial automation, digitization, and the demand for reliable asset management. As of 2025, regulatory and standards bodies such as the American Society of Mechanical Engineers (ASME) and the Institute of Electrical and Electronics Engineers (IEEE) play pivotal roles in shaping best practices and technical guidelines across industries reliant on radial load roller bearings, including manufacturing, energy, transportation, and heavy machinery.
ASME continues to maintain and update foundational codes and standards relevant to bearing design, performance, and diagnostics. Recent years have seen an increased focus on smart monitoring and predictive maintenance. ASME’s standards, such as those covering the operation and maintenance of rotating equipment (e.g., ASME B73 and ASME OM codes), now incorporate recommendations on digital condition monitoring, vibration analysis, and fault detection techniques that are critical for diagnosing issues in radial load roller bearings. These recommendations are being updated to accommodate advancements in sensor technology and data analytics, and to support compliance with stringent safety and reliability requirements for critical infrastructure.
IEEE, meanwhile, is at the forefront of integrating digital diagnostics and data-driven maintenance protocols into electrical and mechanical systems. IEEE standards (e.g., IEEE 841 for electric motors and associated bearing diagnostics) are being revised to reflect the growing adoption of Industrial Internet of Things (IIoT) and machine learning algorithms in condition-based monitoring. These standards help harmonize data formats, communication protocols, and cybersecurity requirements, ensuring that diagnostic tools for radial load roller bearings can operate reliably within increasingly interconnected environments.
Both ASME and IEEE are collaborating internationally to align standards with ISO guidelines, reducing fragmentation and supporting global interoperability. Industry stakeholders, including bearing manufacturers, end-users, and digital solution providers, are participating in working groups to address challenges such as real-time data sharing, standardized diagnostic thresholds, and certification of predictive maintenance systems.
- ASME: Continues to update codes for operation and maintenance of rotating equipment, integrating digital diagnostics.
- IEEE: Revises standards for electric motor and bearing condition monitoring, emphasizing IIoT and cybersecurity.
- Outlook: Expect further convergence of mechanical and digital standards, more open data protocols, and formalization of AI-driven diagnostic acceptance criteria by 2027.
In summary, the regulatory and standards landscape for radial load roller bearing diagnostics is rapidly adapting to technological advances and industrial needs. This trend is expected to accelerate, with ASME and IEEE at the core of regulatory harmonization and the development of robust, future-proof diagnostic frameworks.
Competitive Analysis: Innovations and Patent Activity
The competitive landscape for radial load roller bearing diagnostics in 2025 is characterized by active innovation, with leading bearing manufacturers investing significantly in smart technologies and condition monitoring systems. The top players—such as SKF, Schaeffler Group, NTN Corporation, The Timken Company, and JTEKT Corporation—have accelerated their focus on digitalization, integrating sensors, connectivity, and predictive analytics into bearing solutions.
Patent activity in the diagnostics of radial load roller bearings has increased, with companies seeking to protect innovations in smart sensor integration and data-driven maintenance. For instance, SKF has a strong patent portfolio around wireless sensor platforms, vibration analysis, and machine learning algorithms for early fault detection. Recent patent filings emphasize improvements in real-time monitoring and edge computing for diagnostics, aiming to reduce downtime and improve the reliability of industrial assets.
Similarly, Schaeffler Group has expanded its IP in the realm of digital condition monitoring, especially for applications in wind energy and railways. The company’s strategic collaborations with automation and IoT firms are enabling advanced diagnostic features—such as cloud-based analytics and remote health assessment—for radial load bearings in critical infrastructure. The Timken Company is also actively patenting new sensorized bearing assemblies and data analytics models, aiming to enhance predictive maintenance capabilities for both OEMs and end-users.
In Asia, NTN Corporation and JTEKT Corporation are investing in proprietary sensor technologies and AI-driven diagnostic software. Their patent activities frequently target high-speed and heavy-load applications, reflecting regional demand in automotive, steel, and industrial automation sectors. Notably, NTN’s recent innovation in compact sensor modules for radial bearings has gained attention for its integration flexibility across diverse machinery.
Looking ahead, the next few years are expected to see increased competition over the digitization of bearing diagnostics. Global standards for data interoperability and cybersecurity are likely to shape patent strategies and product development. As industrial operators prioritize uptime and asset health, manufacturers with robust IP in diagnostics and analytics are poised to capture greater market share, especially as Industry 4.0 initiatives accelerate worldwide.
Challenges and Barriers to Adoption
The adoption of advanced diagnostics for radial load roller bearings faces a complex array of challenges and barriers, even as condition monitoring technologies mature and the value of predictive maintenance becomes increasingly clear across industrial sectors. In 2025, several key factors continue to influence the pace and effectiveness of deployment.
One significant challenge is the integration of diagnostic systems into legacy equipment. Many industrial facilities operate with machinery designed long before the advent of modern sensor technologies and digital connectivity. Retrofitting such equipment with vibration, temperature, or acoustic emission sensors often requires custom engineering, driving up both initial costs and installation times. Even when integration is technically feasible, compatibility with existing controls and data platforms remains a persistent hurdle, as many legacy programmable logic controllers (PLCs) and supervisory control and data acquisition (SCADA) systems lack support for advanced analytics or cloud connectivity.
Data quality and interpretation present further obstacles. While sensor costs have declined and edge computing capabilities have improved, ensuring accurate, reliable data collection in harsh or variable environments is nontrivial. Contaminants, fluctuating loads, and electromagnetic interference can all degrade sensor performance. Moreover, diagnostic algorithms frequently require large datasets for effective machine learning, yet operational data from real-world plants is often incomplete, inconsistent, or poorly labeled. This complicates both the training and validation of predictive models for bearing failure.
Workforce skills shortages exacerbate these technological challenges. Implementing and maintaining diagnostic systems requires expertise in both bearing mechanics and digital analytics—skills that remain in short supply across many industries. Training programs and vendor support services are expanding, but the gap in skilled personnel is not expected to close rapidly. Industry leaders such as SKF and The Timken Company have responded by offering integrated monitoring solutions and remote diagnostic services, but widespread in-house adoption is still hampered by the need for specialized knowledge.
Cybersecurity and data privacy represent emerging concerns as more bearing diagnostic systems become networked and cloud-enabled. Securing sensitive operational data from cyber threats is now a prerequisite for industrial adoption, particularly in sectors such as energy, transportation, and critical infrastructure. Companies like Schaeffler Group and NTN Corporation are investing in secure IoT platforms and encrypted data transmission, but regulatory standards and best practices are still evolving.
Looking forward, overcoming these barriers will require coordinated advances in sensor integration, data management, workforce development, and cybersecurity. While leading bearing manufacturers and automation suppliers are making substantial progress, broader adoption will depend on open standards, interoperable technologies, and sustained investment in skills and infrastructure.
Future Outlook: Disruptive Opportunities and Investment Hotspots
The future outlook for radial load roller bearing diagnostics in 2025 and the subsequent years is shaped by disruptive technological advancements, rising industrial automation, and expanding investment in predictive maintenance solutions. The convergence of condition monitoring sensors, artificial intelligence (AI), and Industrial Internet of Things (IIoT) platforms is creating new opportunities for early fault detection, reducing unplanned downtime and optimizing asset lifecycle management.
A key driver is the rapid adoption of smart bearings equipped with embedded sensors capable of monitoring parameters such as vibration, temperature, and load in real time. Leading bearing manufacturers, including Schaeffler AG and SKF Group, are deploying integrated sensor solutions to offer predictive analytics and remote diagnostics. For instance, SKF Group has rolled out cloud-connected bearing solutions that leverage machine learning to identify potential failures before they occur, directly addressing the needs of sectors such as wind energy, rail, and heavy manufacturing.
Another opportunity is the expansion of digital monitoring platforms. The Timken Company, known for its expertise in roller bearing manufacturing, is investing in advanced analytics and digital service offerings, enabling customers to access diagnostics data across global operations. This trend is mirrored by Schaeffler AG, which has established digital condition monitoring centers providing real-time insights and maintenance recommendations.
Investment hotspots are emerging in industries where unplanned downtime is particularly costly, such as aerospace, automotive, and renewable energy. In these sectors, the deployment of automated diagnostic systems is accelerating, supported by partnerships between bearing manufacturers and automation technology firms. For example, SKF Group collaborates with industrial automation leaders to integrate bearing diagnostics into broader asset management platforms.
Looking toward the next few years, disruptive opportunities are anticipated in the development of self-learning diagnostic algorithms and edge computing devices capable of processing sensor data locally. The increasing prevalence of open-data architectures will also foster interoperability between diagnostic systems and existing plant management infrastructure. As a result, investment is expected to concentrate on R&D in smart sensor technology, AI-driven analytics, and scalable digital platforms.
In summary, the radial load roller bearing diagnostics sector is poised for significant transformation, driven by smart technology adoption, digitalization, and cross-industry collaboration. The companies leading this charge—such as SKF Group, Schaeffler AG, and The Timken Company—are setting benchmarks for innovation and investment focus as the market evolves through 2025 and beyond.
Sources & References
- SKF
- Schaeffler
- NSK Ltd.
- Timken
- JTEKT Corporation
- ISO
- ANSI
- American Society of Mechanical Engineers (ASME)
- Institute of Electrical and Electronics Engineers (IEEE)