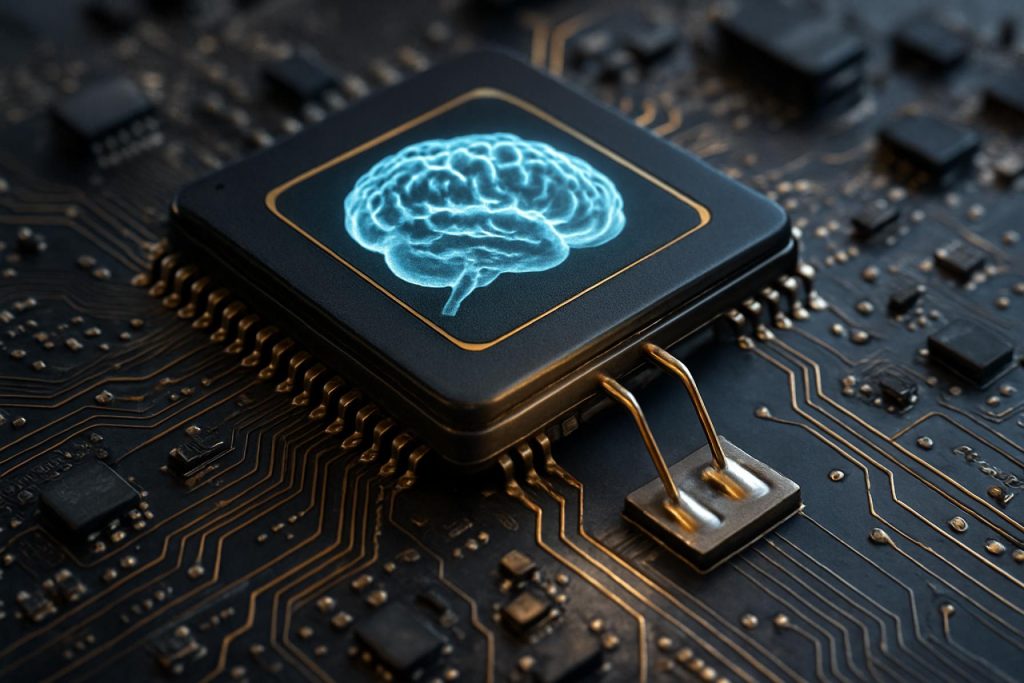
Memristor-Based Neuromorphic Engineering in 2025: Unleashing the Next Wave of Ultra-Efficient, Brain-Like Computing. Explore How Memristors Are Transforming AI Hardware and Shaping the Future of Intelligent Systems.
- Executive Summary: The 2025 Landscape of Memristor Neuromorphic Engineering
- Technology Fundamentals: How Memristors Enable Brain-Inspired Computing
- Key Industry Players and Ecosystem Overview
- Recent Breakthroughs and Prototypes (2023–2025)
- Market Size, Growth Projections, and Investment Trends (2025–2030)
- Application Spotlight: AI, Edge Computing, and Robotics
- Manufacturing Challenges and Supply Chain Dynamics
- Regulatory, Standardization, and Industry Initiatives
- Competitive Analysis: Memristors vs. Traditional and Emerging AI Hardware
- Future Outlook: Roadmap to Commercialization and Long-Term Impact
- Sources & References
Executive Summary: The 2025 Landscape of Memristor Neuromorphic Engineering
Memristor-based neuromorphic engineering is poised for significant advancements in 2025, driven by the convergence of materials innovation, device miniaturization, and the urgent demand for energy-efficient artificial intelligence (AI) hardware. Memristors—resistive switching devices that emulate synaptic behavior—are increasingly recognized as foundational components for next-generation neuromorphic systems, which aim to replicate the parallelism and adaptability of biological neural networks.
In 2025, several leading semiconductor and electronics companies are accelerating the commercialization of memristor technologies. HP Inc. continues to be a pioneer, leveraging its early patents and research in memristive systems to develop scalable crossbar arrays for in-memory computing. Samsung Electronics has announced progress in integrating oxide-based memristors into neuromorphic chips, targeting edge AI applications that require low power consumption and real-time learning. Taiwan Semiconductor Manufacturing Company (TSMC) is collaborating with academic and industrial partners to explore memristor fabrication at advanced process nodes, aiming to bridge the gap between research prototypes and mass production.
Recent demonstrations have shown memristor arrays achieving synaptic densities exceeding 1010 devices per square centimeter, with switching energies in the femtojoule range—orders of magnitude lower than conventional CMOS-based synapses. These advances are enabling neuromorphic processors that can perform complex pattern recognition and adaptive control tasks with a fraction of the energy and latency of traditional von Neumann architectures.
The ecosystem is also expanding beyond hardware. Intel Corporation is investing in software frameworks and development tools tailored for memristor-based neuromorphic platforms, facilitating the deployment of spiking neural networks and event-driven AI models. Meanwhile, imec, a leading nanoelectronics research center, is spearheading collaborative projects to standardize memristor device characterization and benchmarking, which is critical for industry-wide adoption.
Looking ahead, the next few years are expected to see the first commercial deployments of memristor-based neuromorphic chips in edge devices, autonomous systems, and industrial IoT. The sector faces challenges in device variability, endurance, and large-scale integration, but ongoing investments and cross-sector partnerships are accelerating solutions. As the technology matures, memristor-based neuromorphic engineering is set to redefine the landscape of AI hardware, offering unprecedented efficiency and cognitive capabilities for a wide range of applications.
Technology Fundamentals: How Memristors Enable Brain-Inspired Computing
Memristor-based neuromorphic engineering is rapidly advancing as a foundational technology for brain-inspired computing, leveraging the unique properties of memristors—resistive switching devices that retain memory of past electrical states. Unlike traditional CMOS transistors, memristors can emulate synaptic plasticity, a key feature of biological neural networks, by modulating their conductance in response to electrical stimuli. This enables the creation of dense, energy-efficient hardware capable of parallel information processing, a hallmark of the human brain.
In 2025, several industry leaders and research institutions are accelerating the development and commercialization of memristor-based neuromorphic systems. HP Inc. has been a pioneer in memristor research, with ongoing efforts to integrate memristive devices into scalable crossbar arrays for neuromorphic processors. Their work focuses on achieving high device uniformity and endurance, critical for reliable large-scale deployment. Similarly, Samsung Electronics is actively developing memristor arrays for in-memory computing, targeting applications in artificial intelligence (AI) and edge computing. Samsung’s research highlights the potential for memristor-based hardware to dramatically reduce power consumption and latency compared to conventional von Neumann architectures.
European initiatives are also prominent, with Infineon Technologies and STMicroelectronics participating in collaborative projects to advance memristor fabrication and integration. These companies are exploring hybrid CMOS-memristor circuits to enhance the scalability and functionality of neuromorphic chips. In parallel, imec, a leading nanoelectronics research center, is working on optimizing the materials and device structures for memristors, aiming to improve switching speed, retention, and compatibility with existing semiconductor processes.
Recent demonstrations have shown memristor-based synaptic arrays performing unsupervised learning tasks, such as pattern recognition and associative memory, with high efficiency. For example, prototype chips have achieved on-chip learning with power consumption orders of magnitude lower than digital counterparts. The next few years are expected to see the first commercial deployments of memristor-based neuromorphic accelerators in edge devices, IoT sensors, and robotics, where low-power, real-time processing is essential.
Looking ahead, the outlook for memristor-based neuromorphic engineering is promising. As fabrication techniques mature and device variability is further reduced, industry experts anticipate broader adoption in AI hardware, autonomous systems, and adaptive control applications. The convergence of materials innovation, circuit design, and system integration is set to position memristors as a cornerstone of next-generation computing architectures, bridging the gap between biological intelligence and silicon-based machines.
Key Industry Players and Ecosystem Overview
The memristor-based neuromorphic engineering sector in 2025 is characterized by a dynamic ecosystem of semiconductor manufacturers, research-driven startups, and established technology conglomerates. These entities are collectively advancing the commercialization and integration of memristor technology into neuromorphic computing platforms, targeting applications in artificial intelligence (AI), edge computing, and next-generation memory systems.
Among the most prominent industry players is HP Inc., which has been a pioneer in memristor research since the late 2000s. HP’s continued investment in memristor-based architectures underpins its strategy for energy-efficient, high-density memory and neuromorphic processors. The company’s collaborations with academic and industrial partners have resulted in several prototype systems, with ongoing efforts to scale production for commercial deployment.
Another key participant is Samsung Electronics, leveraging its leadership in semiconductor fabrication to explore memristor arrays for AI accelerators and in-memory computing. Samsung’s research divisions have demonstrated high-density memristor crossbar arrays and are actively working on integrating these devices into their next-generation memory and logic products. The company’s roadmap suggests pilot deployments in edge AI hardware within the next few years.
In Europe, Infineon Technologies is notable for its focus on neuromorphic hardware for automotive and industrial automation. Infineon’s R&D initiatives include the development of memristor-based synaptic arrays, aiming to enhance real-time data processing and reduce power consumption in embedded systems. The company collaborates with European research consortia to accelerate the transition from laboratory prototypes to market-ready solutions.
Startups and university spin-offs also play a vital role in the ecosystem. Companies such as Crossbar Inc. are commercializing resistive RAM (ReRAM) technologies, which are closely related to memristors and form the basis for several neuromorphic computing prototypes. Crossbar’s technology is being evaluated for integration into AI inference chips and low-power IoT devices.
The ecosystem is further supported by foundries and materials suppliers, including TSMC, which provides advanced process nodes for fabricating memristor arrays, and by collaborative initiatives with academic institutions and government agencies. These partnerships are crucial for addressing challenges such as device variability, scalability, and integration with CMOS technology.
Looking ahead, the next few years are expected to see increased pilot deployments and early commercial products, particularly in edge AI and sensor fusion applications. The convergence of expertise from established semiconductor leaders, innovative startups, and research organizations is poised to accelerate the adoption of memristor-based neuromorphic systems, with significant implications for the broader AI hardware landscape.
Recent Breakthroughs and Prototypes (2023–2025)
Between 2023 and 2025, memristor-based neuromorphic engineering has witnessed significant breakthroughs, with several prototypes and demonstrators emerging from both established semiconductor companies and specialized startups. These advances are driven by the need for energy-efficient, brain-inspired computing architectures capable of handling AI workloads beyond the reach of conventional CMOS technology.
One of the most notable developments is the demonstration of large-scale memristor arrays integrated with CMOS circuits, enabling in-memory computing for neural network acceleration. SK hynix, a major memory manufacturer, has publicly announced research into memristive devices for neuromorphic applications, focusing on resistive RAM (ReRAM) arrays that mimic synaptic behavior. Their prototypes have shown promising endurance and retention characteristics, essential for practical deployment in edge AI systems.
Similarly, Samsung Electronics has reported progress in developing memristor-based crossbar arrays, targeting ultra-low-power AI inference engines. In 2024, Samsung demonstrated a prototype neuromorphic chip integrating over 100,000 memristive synapses, achieving real-time pattern recognition with a fraction of the energy required by traditional digital accelerators. This work aligns with Samsung’s broader strategy to lead in next-generation memory and AI hardware.
On the startup front, TSMC—while primarily a foundry—has collaborated with academic and industrial partners to fabricate memristor test chips using advanced process nodes, enabling rapid prototyping and scaling studies. These collaborations have resulted in demonstration chips that combine memristive crossbars with digital logic, paving the way for hybrid neuromorphic processors.
In Europe, Infineon Technologies has invested in research consortia focused on memristor-based neuromorphic hardware for automotive and industrial IoT. Their recent prototypes emphasize robustness and reliability, addressing the stringent requirements of embedded AI in safety-critical environments.
Looking ahead to 2025 and beyond, the outlook for memristor-based neuromorphic engineering is increasingly optimistic. Industry roadmaps suggest that commercial pilot deployments in edge AI, robotics, and sensor fusion could emerge within the next few years, leveraging the unique advantages of memristive devices: non-volatility, analog programmability, and high integration density. As fabrication processes mature and device variability is further reduced, memristor-based neuromorphic chips are poised to become a cornerstone of next-generation AI hardware platforms.
Market Size, Growth Projections, and Investment Trends (2025–2030)
The market for memristor-based neuromorphic engineering is poised for significant expansion between 2025 and 2030, driven by escalating demand for energy-efficient, brain-inspired computing in artificial intelligence (AI), edge devices, and next-generation memory systems. As of 2025, the sector is transitioning from research and prototyping to early-stage commercialization, with several leading semiconductor manufacturers and technology firms investing in the development and scaling of memristor technologies.
Key industry players such as HP Inc. and Samsung Electronics have been at the forefront of memristor research, with HP pioneering the first practical memristor device and Samsung actively exploring resistive RAM (ReRAM) and neuromorphic hardware. Taiwan Semiconductor Manufacturing Company (TSMC) and Intel Corporation are also investing in advanced memory and neuromorphic chip architectures, leveraging their foundry capabilities and AI hardware expertise. These companies are expected to accelerate pilot production and integration of memristor arrays into neuromorphic processors and memory modules over the next few years.
The market size for memristor-based neuromorphic systems is projected to grow at a compound annual growth rate (CAGR) exceeding 30% through 2030, as indicated by industry roadmaps and public statements from major manufacturers. This growth is fueled by the increasing adoption of edge AI, autonomous vehicles, robotics, and IoT devices, all of which benefit from the low-power, high-density, and analog computation capabilities of memristor-based hardware. For example, Sony Group Corporation has announced ongoing research into neuromorphic vision sensors, which may incorporate memristive elements for on-sensor processing.
Investment trends in 2025 reflect a surge in both corporate R&D and venture capital funding targeting startups and university spin-offs specializing in memristor fabrication, materials, and neuromorphic algorithms. Notable collaborations include partnerships between established semiconductor firms and academic institutions to accelerate the translation of laboratory breakthroughs into manufacturable products. IBM continues to invest in neuromorphic computing research, with a focus on integrating memristive devices into scalable AI accelerators.
Looking ahead, the outlook for 2025–2030 suggests that memristor-based neuromorphic engineering will move from niche applications to broader deployment in commercial AI hardware, particularly as manufacturing yields improve and standardization efforts mature. The sector is expected to attract sustained investment, with leading companies expanding pilot lines and forming strategic alliances to capture emerging opportunities in AI, edge computing, and next-generation memory markets.
Application Spotlight: AI, Edge Computing, and Robotics
Memristor-based neuromorphic engineering is rapidly advancing as a transformative technology for AI, edge computing, and robotics, with 2025 marking a pivotal year for both research breakthroughs and early-stage commercialization. Memristors—resistive switching devices that mimic synaptic behavior—are enabling hardware architectures that process information in ways analogous to the human brain, offering significant improvements in energy efficiency, speed, and scalability over traditional CMOS-based systems.
In AI, memristor arrays are being integrated into neuromorphic chips to accelerate deep learning inference and on-chip training. Companies such as SK hynix and Samsung Electronics have publicly demonstrated prototype memristor-based memory and processing units, targeting AI workloads that require low latency and high throughput. These devices leverage analog computation within crossbar arrays, reducing the need for data movement and thus slashing power consumption—a critical advantage for edge AI applications.
Edge computing is another area where memristor-based neuromorphic hardware is gaining traction. The ability to perform complex AI tasks locally, without reliance on cloud infrastructure, is essential for applications such as autonomous vehicles, industrial IoT, and smart sensors. Huawei has announced ongoing research into memristor-based accelerators for edge devices, aiming to deliver real-time processing with minimal energy requirements. Similarly, TSMC is collaborating with academic and industry partners to develop fabrication processes optimized for large-scale memristor integration, signaling a move toward manufacturability at commercial volumes.
In robotics, memristor-based neuromorphic systems are being explored for adaptive control, sensor fusion, and real-time decision-making. The non-volatile and analog nature of memristors allows for the implementation of spiking neural networks (SNNs) that can learn and adapt on the fly, closely emulating biological neural circuits. Intel has highlighted memristor research as a key component of its neuromorphic computing roadmap, with the goal of enabling robots that can operate autonomously in dynamic environments.
Looking ahead, the next few years are expected to see the first commercial deployments of memristor-based neuromorphic chips in specialized AI and edge computing products. Challenges remain in terms of device variability, endurance, and large-scale integration, but ongoing investment from major semiconductor manufacturers and research institutions is accelerating progress. As these hurdles are addressed, memristor-based neuromorphic engineering is poised to become a foundational technology for the next generation of intelligent systems.
Manufacturing Challenges and Supply Chain Dynamics
The manufacturing landscape for memristor-based neuromorphic engineering in 2025 is characterized by both rapid innovation and significant hurdles. As memristors transition from laboratory prototypes to commercial-scale production, several challenges in fabrication, materials sourcing, and supply chain integration have become increasingly prominent.
One of the primary manufacturing challenges is the precise control of nanoscale features required for memristor devices. Unlike conventional CMOS technology, memristors often rely on complex oxide materials and atomic-scale switching mechanisms, demanding advanced deposition and patterning techniques. Leading semiconductor foundries such as Taiwan Semiconductor Manufacturing Company (TSMC) and Samsung Electronics have begun exploring pilot lines for memristive devices, leveraging their expertise in extreme ultraviolet (EUV) lithography and atomic layer deposition. However, yield variability and device uniformity remain persistent issues, especially as device densities increase for neuromorphic applications.
Material supply is another critical factor. Memristors often utilize transition metal oxides (e.g., HfO2, TiO2), silver, or other rare elements. The sourcing of high-purity materials at scale is complicated by global supply chain disruptions and geopolitical tensions, particularly for metals and rare earths. Companies like Infineon Technologies and STMicroelectronics are actively working to secure diversified supply chains and develop alternative material systems to mitigate these risks.
Integration with existing semiconductor manufacturing infrastructure is another bottleneck. Memristor fabrication often requires process steps incompatible with standard CMOS lines, necessitating either dedicated facilities or costly retrofitting. Collaborative efforts between device innovators and foundries are underway to develop hybrid process flows. For example, HP Inc., a pioneer in memristor research, has partnered with manufacturing specialists to adapt memristor integration for neuromorphic chips, focusing on scalable crossbar array architectures.
On the supply chain front, the global semiconductor shortage of the early 2020s has prompted a reevaluation of logistics and inventory strategies. Companies are increasingly adopting multi-sourcing and regional manufacturing models to enhance resilience. The emergence of specialized suppliers for memristor materials and equipment is expected to accelerate over the next few years, with industry consortia and standards bodies playing a key role in harmonizing specifications and quality controls.
Looking ahead, the outlook for memristor-based neuromorphic engineering hinges on overcoming these manufacturing and supply chain challenges. As pilot production lines mature and ecosystem partnerships deepen, the sector is poised for incremental but steady progress toward commercial viability, with the potential for broader adoption in edge AI, robotics, and next-generation computing platforms by the late 2020s.
Regulatory, Standardization, and Industry Initiatives
The regulatory and standardization landscape for memristor-based neuromorphic engineering is rapidly evolving as the technology matures and moves closer to commercial deployment. In 2025, industry stakeholders are increasingly collaborating to establish frameworks that ensure interoperability, safety, and reliability of memristor-enabled systems, particularly as these devices are poised to play a critical role in next-generation artificial intelligence (AI) hardware.
A key driver in this space is the Institute of Electrical and Electronics Engineers (IEEE), which has initiated working groups focused on standards for neuromorphic computing architectures, including those leveraging memristive devices. The IEEE P2846 and P2020 standards, while originally focused on AI and autonomous systems, are being expanded to address the unique requirements of memristor-based hardware, such as device variability, endurance, and integration with CMOS technologies. These efforts are expected to yield draft standards by late 2025, providing a foundation for industry-wide adoption.
On the regulatory front, agencies such as the National Institute of Standards and Technology (NIST) in the United States are actively engaging with industry leaders to develop measurement protocols and reliability benchmarks for memristive devices. NIST’s involvement is crucial for establishing trust in the performance and long-term stability of memristor-based neuromorphic chips, especially for safety-critical applications in automotive and healthcare sectors.
Industry consortia are also playing a pivotal role. The SEMI association, representing the global electronics manufacturing supply chain, has launched initiatives to harmonize process standards for emerging memory technologies, including memristors. These initiatives aim to streamline manufacturing, testing, and quality assurance processes, facilitating the transition from laboratory prototypes to scalable commercial products.
Leading companies such as HP Inc. and Samsung Electronics are actively participating in these standardization efforts. HP, a pioneer in memristor research, is contributing to the development of device-level standards and sharing best practices from its own R&D programs. Samsung, with its extensive experience in memory manufacturing, is focusing on integration challenges and reliability metrics, leveraging its global manufacturing footprint to influence industry-wide practices.
Looking ahead, the next few years are expected to see the formalization of international standards and regulatory guidelines, which will be instrumental in accelerating the adoption of memristor-based neuromorphic systems. As these frameworks mature, they will provide a clear pathway for certification, interoperability, and cross-industry collaboration, ultimately enabling broader deployment of energy-efficient, brain-inspired computing platforms.
Competitive Analysis: Memristors vs. Traditional and Emerging AI Hardware
The competitive landscape for memristor-based neuromorphic engineering in 2025 is defined by rapid technological advances, intensifying competition with both traditional and emerging AI hardware paradigms, and a growing ecosystem of industry players. Memristors—resistive switching devices that mimic synaptic behavior—are increasingly positioned as a disruptive alternative to conventional CMOS-based digital processors and even to specialized AI accelerators such as GPUs, TPUs, and FPGAs.
Traditional AI hardware, dominated by companies like Intel and NVIDIA, relies on von Neumann architectures, which separate memory and processing units. This separation leads to the so-called “memory wall,” limiting energy efficiency and speed, especially in data-intensive neural network workloads. In contrast, memristor-based neuromorphic systems integrate memory and computation at the device level, enabling in-memory processing and massively parallel architectures that closely emulate biological neural networks.
In 2025, several companies are actively developing and commercializing memristor-based solutions. HP Inc. has been a pioneer, with its long-term research into memristive devices and the development of the “The Machine” architecture, which leverages memristors for high-speed, energy-efficient computing. Samsung Electronics is also investing in memristor research, focusing on resistive RAM (ReRAM) and its integration into neuromorphic chips for edge AI applications. TSMC, the world’s largest semiconductor foundry, is collaborating with academic and industrial partners to develop scalable memristor fabrication processes, aiming to bring these devices to commercial viability.
Emerging AI hardware, such as photonic processors and quantum accelerators, presents additional competition. However, memristor-based neuromorphic chips offer unique advantages in terms of ultra-low power consumption, high density, and the ability to perform analog computation, which is particularly suited for spiking neural networks and real-time sensory processing. Early prototypes have demonstrated orders-of-magnitude improvements in energy efficiency compared to digital AI accelerators, with some memristor arrays achieving sub-picojoule per synaptic operation.
Looking ahead, the next few years are expected to see further convergence between memristor technology and advanced AI workloads, particularly in edge computing, robotics, and autonomous systems. Industry roadmaps suggest that by 2027, memristor-based neuromorphic chips could begin to see broader adoption in commercial products, provided that challenges related to device variability, large-scale integration, and standardization are addressed. The competitive dynamics will hinge on the ability of memristor developers to scale manufacturing, ensure reliability, and demonstrate clear performance gains over both traditional and other emerging AI hardware solutions.
Future Outlook: Roadmap to Commercialization and Long-Term Impact
The future outlook for memristor-based neuromorphic engineering in 2025 and the coming years is marked by a transition from laboratory prototypes to early-stage commercial deployments, with significant implications for computing, artificial intelligence, and edge devices. As the demand for energy-efficient, brain-inspired hardware accelerates, memristor technology is positioned to address the limitations of conventional CMOS-based architectures, particularly in terms of power consumption, parallelism, and scalability.
Several leading semiconductor and electronics companies are actively advancing memristor-based neuromorphic platforms. HP Inc. has been a pioneer in memristor research, with ongoing efforts to integrate memristive devices into memory and logic circuits for neuromorphic applications. Samsung Electronics is also investing in next-generation memory technologies, including memristors, to enable more efficient AI accelerators and edge computing solutions. Taiwan Semiconductor Manufacturing Company (TSMC), as the world’s largest contract chipmaker, is collaborating with research institutions to explore the integration of memristive elements into advanced process nodes, aiming for manufacturability and scalability.
In 2025, the industry is expected to see the first commercial demonstrations of memristor-based neuromorphic chips targeting niche markets such as low-power IoT sensors, real-time pattern recognition, and adaptive robotics. These early products will likely leverage the analog computing capabilities of memristors to perform in-memory processing, reducing latency and energy requirements compared to traditional von Neumann architectures. For example, SK hynix and Micron Technology are both exploring resistive RAM (ReRAM) and related memristive technologies as enablers for neuromorphic hardware, with pilot projects and prototype chips anticipated in the near term.
Despite these advances, several challenges remain on the roadmap to widespread commercialization. Key technical hurdles include device variability, endurance, and large-scale integration with existing CMOS processes. Industry consortia and standardization bodies, such as the SEMI and the IEEE, are working to establish interoperability standards and best practices for memristor-based systems, which will be critical for ecosystem development and supply chain readiness.
Looking ahead, the long-term impact of memristor-based neuromorphic engineering could be transformative. As manufacturing yields improve and integration challenges are addressed, these technologies are expected to enable ultra-low-power, adaptive computing platforms for edge AI, autonomous systems, and next-generation data centers. The next few years will be pivotal, as industry leaders move from proof-of-concept to scalable products, setting the stage for broader adoption and a paradigm shift in how intelligent systems are designed and deployed.
Sources & References
- imec
- Infineon Technologies
- STMicroelectronics
- Crossbar Inc.
- IBM
- Huawei
- Institute of Electrical and Electronics Engineers (IEEE)
- National Institute of Standards and Technology (NIST)
- NVIDIA
- Micron Technology