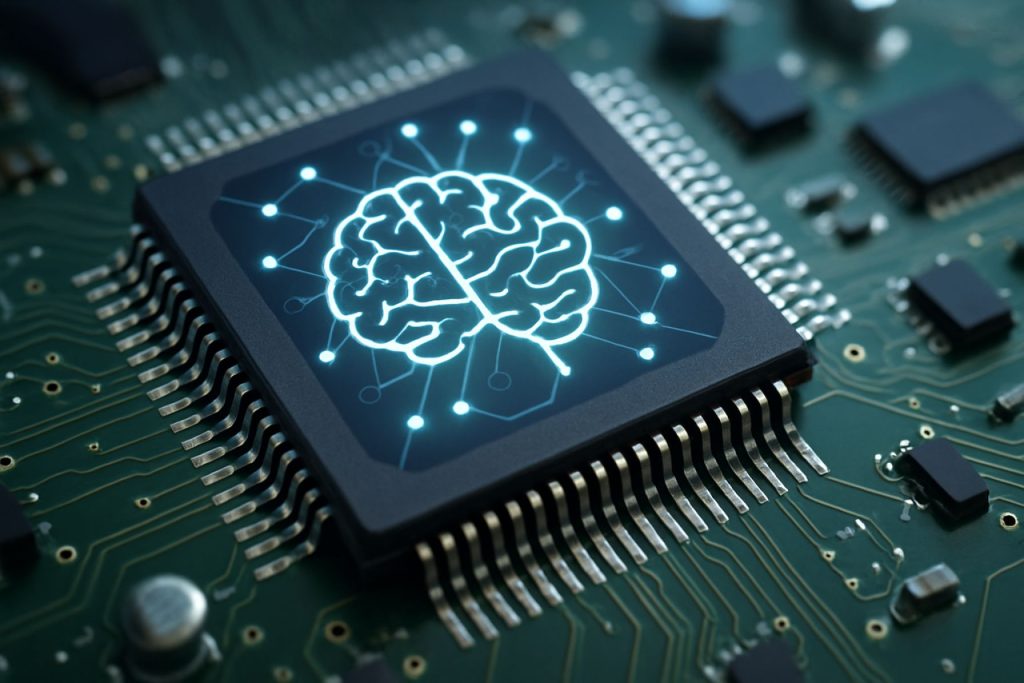
Device-Level Neuromorphic Computing Systems in 2025: Unleashing the Next Wave of AI Hardware Innovation. Explore How Emerging Architectures and Materials Will Transform Edge Intelligence Over the Next Five Years.
- Executive Summary: 2025 Market Outlook and Key Drivers
- Technology Landscape: Core Architectures and Device Innovations
- Material Advances: Memristors, Phase-Change, and Beyond
- Leading Players and Strategic Initiatives (e.g., intel.com, ibm.com, synsense.com)
- Current Market Size, Segmentation, and 2025–2030 CAGR Analysis (Est. 30%+ Growth)
- Application Frontiers: Edge AI, Robotics, IoT, and Autonomous Systems
- Challenges: Scalability, Standardization, and Integration Barriers
- Regulatory and Industry Standards (e.g., ieee.org, neuromorphicsociety.org)
- Investment Trends and Funding Landscape
- Future Outlook: Disruptive Potential and Long-Term Impact on AI Hardware
- Sources & References
Executive Summary: 2025 Market Outlook and Key Drivers
Device-level neuromorphic computing systems are poised for significant advancements and market momentum in 2025, driven by breakthroughs in materials, architectures, and integration with edge AI applications. These systems, inspired by the structure and function of biological neural networks, leverage novel devices such as memristors, phase-change memory, and spintronic elements to enable highly efficient, low-power computation. The year 2025 is expected to see a transition from research prototypes to early-stage commercial deployments, particularly in sectors demanding real-time, energy-efficient processing such as autonomous vehicles, robotics, and IoT edge devices.
Key industry players are accelerating the development and scaling of neuromorphic hardware. Intel Corporation continues to advance its Loihi neuromorphic research chip, focusing on scalability and integration with conventional AI workflows. IBM is also active in this space, leveraging its expertise in phase-change memory and brain-inspired architectures to push the boundaries of device-level performance. Meanwhile, Samsung Electronics and Toshiba Corporation are exploring the commercialization of memristor-based and other non-volatile memory technologies for neuromorphic applications, aiming to bridge the gap between research and mass production.
In 2025, the market outlook is shaped by several key drivers:
- Edge AI Demand: The proliferation of edge devices requiring real-time, low-latency inference is accelerating the adoption of neuromorphic hardware, which offers significant power and speed advantages over traditional von Neumann architectures.
- Material and Device Innovations: Advances in materials science, such as the development of more reliable and scalable memristors and phase-change devices, are enabling higher-density, more robust neuromorphic chips.
- Collaborative Ecosystems: Partnerships between hardware manufacturers, research institutions, and software developers are fostering the creation of standardized platforms and development tools, lowering barriers to entry for new applications.
- Government and Industry Investment: Increased funding from both public and private sectors is accelerating R&D and pilot projects, particularly in the US, Europe, and East Asia.
Looking ahead, the next few years are expected to witness the emergence of commercial neuromorphic processors in niche markets, with broader adoption contingent on further improvements in device reliability, programmability, and integration with existing AI ecosystems. As leading companies such as Intel Corporation, IBM, Samsung Electronics, and Toshiba Corporation continue to invest in device-level innovation, the sector is positioned for robust growth and technological breakthroughs through 2025 and beyond.
Technology Landscape: Core Architectures and Device Innovations
Device-level neuromorphic computing systems are at the forefront of a technological shift, aiming to emulate the efficiency and parallelism of biological neural networks directly in hardware. As of 2025, the landscape is defined by rapid advances in both materials and architectures, with a focus on overcoming the limitations of traditional von Neumann computing.
A central trend is the development of novel memory and logic devices that can natively implement synaptic and neuronal functions. Resistive RAM (ReRAM), phase-change memory (PCM), and spintronic devices are among the most prominent candidates. IBM has been a pioneer in phase-change memory-based neuromorphic hardware, demonstrating large-scale arrays capable of in-memory computing and synaptic plasticity. Their research prototypes have shown the feasibility of integrating millions of artificial synapses on a single chip, with ongoing work to improve endurance and energy efficiency.
Similarly, Intel continues to advance its Loihi neuromorphic processor family, which leverages custom digital circuits to mimic spiking neural networks. The latest Loihi 2 chip, released in late 2023, features improved scalability, programmability, and support for on-chip learning, positioning it as a key platform for both academic and industrial research in 2025. Intel’s approach emphasizes flexibility, allowing researchers to experiment with a variety of neuron and synapse models at the device level.
In the realm of emerging materials, Samsung Electronics has made significant investments in oxide-based ReRAM and ferroelectric memory technologies, targeting their integration into neuromorphic accelerators. Their work focuses on achieving high-density, low-power synaptic arrays suitable for edge AI applications. Meanwhile, Taiwan Semiconductor Manufacturing Company (TSMC) is collaborating with academic and industrial partners to develop process technologies that enable the mass production of neuromorphic chips, including 3D integration and advanced packaging.
Looking ahead, the next few years are expected to see the first commercial deployments of device-level neuromorphic systems in specialized domains such as robotics, sensor fusion, and always-on edge inference. The convergence of advances in non-volatile memory, analog computing, and scalable architectures is likely to yield chips that offer orders-of-magnitude improvements in energy efficiency and latency compared to conventional AI accelerators. However, challenges remain in device variability, large-scale integration, and standardization, which industry leaders are actively addressing through cross-sector collaborations and open hardware initiatives.
Material Advances: Memristors, Phase-Change, and Beyond
Device-level neuromorphic computing systems are rapidly evolving, driven by significant advances in materials such as memristors, phase-change memory (PCM), and emerging alternatives. These materials are foundational for emulating synaptic and neuronal functions in hardware, enabling energy-efficient and highly parallel information processing that mimics biological brains.
Memristors, which modulate resistance based on the history of voltage and current, have become a focal point for neuromorphic hardware. In 2025, leading semiconductor manufacturers are scaling up production of memristor arrays with improved endurance and retention. HP Inc. continues to develop its metal-oxide memristor technology, targeting integration into edge AI accelerators and in-memory computing platforms. Meanwhile, Samsung Electronics is leveraging its expertise in advanced memory to prototype large-scale memristor crossbar arrays, aiming for high-density, low-power neuromorphic chips suitable for mobile and IoT applications.
Phase-change memory (PCM) is another promising material system, utilizing the reversible transition between amorphous and crystalline states to store information. Intel Corporation has demonstrated PCM-based synaptic arrays capable of analog weight storage, a key requirement for efficient neural network inference and training. In 2025, Intel is collaborating with academic and industrial partners to optimize PCM devices for lower switching energy and higher cycling endurance, addressing critical barriers to commercial deployment in neuromorphic processors.
Beyond memristors and PCM, ferroelectric and spintronic devices are gaining traction. GlobalFoundries is exploring ferroelectric field-effect transistors (FeFETs) for non-volatile, analog memory elements, which could further reduce power consumption in neuromorphic circuits. Spintronic devices, such as magnetic tunnel junctions (MTJs), are being investigated by IBM for their potential to combine memory and logic in a single device, offering ultra-fast and energy-efficient synaptic operations.
The outlook for device-level neuromorphic systems in the next few years is marked by a transition from laboratory prototypes to early commercial products. Industry consortia and government initiatives are accelerating standardization and ecosystem development, with a focus on reliability, scalability, and integration with conventional CMOS processes. As these material advances mature, neuromorphic hardware is expected to find initial deployment in edge AI, robotics, and sensor fusion, paving the way for broader adoption in data centers and autonomous systems by the late 2020s.
Leading Players and Strategic Initiatives (e.g., intel.com, ibm.com, synsense.com)
Device-level neuromorphic computing systems are rapidly advancing, with several leading technology companies and startups spearheading innovation through dedicated hardware, strategic partnerships, and ecosystem development. As of 2025, the competitive landscape is defined by a mix of established semiconductor giants and specialized neuromorphic firms, each pursuing unique architectures and commercialization strategies.
Intel Corporation remains a prominent force in neuromorphic hardware, building on its Loihi chip family. The Intel Corporation Loihi 2, introduced in late 2021, continues to serve as a research platform for event-driven, spiking neural network (SNN) applications. Intel’s strategic initiatives include collaborations with academic institutions and industry partners to expand the Loihi ecosystem, focusing on real-time robotics, adaptive control, and edge AI. In 2024–2025, Intel is expected to further enhance Loihi’s scalability and energy efficiency, targeting broader adoption in edge devices and autonomous systems.
IBM is another key player, leveraging its long-standing research in brain-inspired computing. The IBM TrueNorth chip, though initially released in 2014, continues to influence IBM’s neuromorphic research. IBM’s current focus is on integrating neuromorphic principles into hybrid AI systems and exploring new device materials for improved performance. Strategic initiatives include partnerships with research consortia and government agencies to accelerate the transition from research prototypes to commercial-grade neuromorphic processors.
In Europe, SynSense (formerly aiCTX) is at the forefront of ultra-low-power neuromorphic hardware. SynSense specializes in event-based vision and audio processing chips, such as the Speck and DYNAP-CNN platforms. In 2025, SynSense is expanding its partnerships with sensor manufacturers and robotics companies to deploy neuromorphic processors in smart cameras, drones, and IoT devices. The company’s strategic direction emphasizes edge intelligence, with a focus on real-time, always-on applications.
Other notable players include BrainChip Holdings, which commercializes the Akida neuromorphic processor for edge AI, and GrAI Matter Labs, which develops low-latency, event-driven chips for robotics and industrial automation. Both companies are actively forming alliances with OEMs and system integrators to accelerate market entry and scale production.
Looking ahead, the next few years will likely see intensified collaboration between hardware developers, software toolchain providers, and end-user industries. Strategic initiatives are expected to focus on standardization, interoperability, and the development of open-source frameworks to lower adoption barriers. As device-level neuromorphic systems mature, their integration into commercial products—particularly in edge computing, autonomous vehicles, and smart sensors—will be a key trend shaping the sector’s outlook through 2025 and beyond.
Current Market Size, Segmentation, and 2025–2030 CAGR Analysis (Est. 30%+ Growth)
Device-level neuromorphic computing systems—hardware platforms that emulate the neural architectures and processing methods of the human brain—are rapidly transitioning from research labs to commercial and industrial applications. As of 2025, the global market for these systems is estimated to be valued at approximately $500 million, with projections indicating a compound annual growth rate (CAGR) exceeding 30% through 2030. This surge is driven by escalating demand for energy-efficient, low-latency AI hardware in edge computing, robotics, autonomous vehicles, and next-generation sensor networks.
The market is segmented by device type, application, and end-user industry. At the device level, the primary categories include analog and digital neuromorphic chips, memristor-based devices, and spintronic components. Analog neuromorphic chips, such as those pioneered by Intel Corporation (notably the Loihi series), are gaining traction for their ability to process spiking neural networks with ultra-low power consumption. Digital neuromorphic platforms, while more mature, are being rapidly iterated to close the efficiency gap. Memristor-based systems, championed by companies like Hewlett Packard Enterprise (HPE) and Samsung Electronics, are at the forefront of non-volatile memory integration, enabling in-memory computing architectures that further reduce energy and latency bottlenecks.
Application-wise, neuromorphic devices are being adopted in edge AI for real-time data processing, industrial automation, and smart sensors. The automotive sector is a key early adopter, leveraging neuromorphic chips for advanced driver-assistance systems (ADAS) and autonomous navigation. Healthcare is another emerging segment, with neuromorphic processors enabling portable diagnostic devices and brain-machine interfaces. Major technology providers such as IBM and Qualcomm Incorporated are actively developing neuromorphic solutions tailored for these verticals.
Geographically, North America and East Asia (notably South Korea and Japan) are leading in both R&D and early commercialization, supported by robust semiconductor ecosystems and government-backed AI initiatives. Europe is also making significant strides, with collaborative projects involving research institutions and industry players.
Looking ahead to 2030, the device-level neuromorphic computing market is expected to surpass $2.2 billion, underpinned by continued advances in materials science, device miniaturization, and integration with conventional CMOS processes. The anticipated CAGR of 30%+ reflects both the nascent stage of the market and the accelerating pace of adoption across diverse sectors. As more companies—ranging from established semiconductor giants to specialized startups—enter the field, competition and innovation are set to intensify, further expanding the market’s scope and impact.
Application Frontiers: Edge AI, Robotics, IoT, and Autonomous Systems
Device-level neuromorphic computing systems are rapidly advancing as foundational enablers for next-generation edge AI, robotics, IoT, and autonomous systems. These systems, inspired by the structure and function of biological neural networks, are designed to process information with high efficiency and low power consumption, making them ideal for deployment in resource-constrained environments.
In 2025, several industry leaders are pushing the boundaries of neuromorphic hardware. Intel Corporation continues to develop its Loihi neuromorphic research chips, which feature digital spiking neural networks capable of on-chip learning and event-driven computation. Loihi’s architecture is particularly suited for real-time sensory processing and adaptive control in robotics and edge devices. Intel has demonstrated Loihi’s ability to perform complex tasks such as gesture recognition and robotic control with orders-of-magnitude lower energy consumption compared to conventional processors.
Similarly, International Business Machines Corporation (IBM) is advancing its TrueNorth chip, which integrates over one million programmable neurons and 256 million synapses. IBM’s neuromorphic systems are being explored for applications in low-power vision, auditory processing, and autonomous navigation, with ongoing collaborations targeting smart sensors and edge analytics.
In the realm of analog and mixed-signal neuromorphic devices, SynSense (formerly aiCTX) is commercializing ultra-low-power neuromorphic processors for always-on sensing in IoT and robotics. Their SNN (spiking neural network) chips are being integrated into smart cameras and edge modules, enabling real-time event-based vision with minimal energy requirements.
Another notable player, BrainChip Holdings Ltd, has introduced the Akida neuromorphic processor, which is being adopted in edge AI applications such as industrial automation, cybersecurity, and autonomous vehicles. Akida’s event-based processing and on-chip learning capabilities allow for rapid adaptation to changing environments, a critical feature for autonomous systems.
Looking ahead, the outlook for device-level neuromorphic computing is robust. The convergence of advances in materials (such as memristive devices), scalable architectures, and software ecosystems is expected to accelerate commercialization. Industry roadmaps indicate that by 2026–2027, neuromorphic chips will be increasingly embedded in edge AI modules, collaborative robots, and distributed IoT nodes, enabling real-time, adaptive intelligence at the device level. As these systems mature, they are poised to transform the efficiency and autonomy of edge computing, paving the way for new frontiers in smart infrastructure, healthcare, and mobility.
Challenges: Scalability, Standardization, and Integration Barriers
Device-level neuromorphic computing systems are at the forefront of next-generation artificial intelligence hardware, but their widespread adoption faces significant challenges in scalability, standardization, and integration. As of 2025, these barriers are shaping the pace and direction of innovation in the sector.
Scalability remains a primary concern. Neuromorphic devices, such as memristors, phase-change memory, and spintronic elements, must be manufactured at scale with high yield and uniformity to be commercially viable. Leading semiconductor manufacturers, including Intel Corporation and Samsung Electronics, have demonstrated prototype neuromorphic chips, but scaling these devices from laboratory to mass production introduces variability in device characteristics, endurance, and retention. For example, Samsung Electronics has reported progress in integrating neuromorphic architectures with their advanced memory technologies, yet acknowledges the challenges in achieving consistent device behavior across large arrays.
Standardization is another critical hurdle. The neuromorphic ecosystem currently lacks unified standards for device interfaces, communication protocols, and benchmarking metrics. This fragmentation impedes interoperability between devices from different vendors and complicates the development of software and tools. Industry consortia and organizations such as the IEEE are beginning to address these gaps by proposing standards for neuromorphic hardware and benchmarking, but widespread adoption is still in its early stages. Without common standards, it is difficult for system integrators and end-users to compare performance or ensure compatibility across platforms.
Integration barriers further complicate the deployment of neuromorphic systems. Device-level neuromorphic components must be seamlessly integrated with conventional CMOS technology and existing digital infrastructure. Companies like IBM and Intel Corporation are actively researching hybrid architectures that combine neuromorphic cores with traditional processors, but challenges remain in terms of power delivery, signal integrity, and packaging. Additionally, the lack of mature design automation tools tailored for neuromorphic circuits slows down the development cycle and increases the risk of design errors.
Looking ahead to the next few years, overcoming these challenges will require coordinated efforts between device manufacturers, standardization bodies, and system integrators. Progress in scalable fabrication, the establishment of industry-wide standards, and advances in heterogeneous integration will be crucial for transitioning neuromorphic computing from research labs to real-world applications.
Regulatory and Industry Standards (e.g., ieee.org, neuromorphicsociety.org)
Device-level neuromorphic computing systems are rapidly advancing, prompting increased attention to regulatory frameworks and industry standards. As of 2025, the field is characterized by a convergence of efforts from international standards organizations, industry consortia, and leading semiconductor manufacturers to ensure interoperability, safety, and scalability of neuromorphic hardware.
The IEEE has been at the forefront of standardization, with its Rebooting Computing Initiative and the IEEE P2846 working group focusing on standards for neuromorphic architectures and their integration into broader computing ecosystems. These efforts aim to define common interfaces, data formats, and performance metrics, which are critical for device-level interoperability and benchmarking. The IEEE is also collaborating with industry partners to address the unique requirements of analog and mixed-signal neuromorphic devices, which differ significantly from conventional digital hardware.
The International Neuromorphic Computing Society (INCS) has emerged as a key player in fostering collaboration between academia, industry, and regulatory bodies. In 2025, the INCS is facilitating working groups to develop best practices for device characterization, reliability testing, and energy efficiency measurement. These guidelines are expected to inform future regulatory policies and certification processes, especially as neuromorphic chips begin to enter safety-critical applications such as autonomous vehicles and medical devices.
Major semiconductor companies, including Intel Corporation and IBM, are actively participating in standards development. Intel’s Loihi 2 chip and IBM’s TrueNorth platform have both served as reference architectures in ongoing discussions about device-level requirements, such as spike-based communication protocols and on-chip learning capabilities. These companies are also engaging with regulatory agencies to ensure that emerging neuromorphic products comply with existing safety and electromagnetic compatibility standards, while advocating for updates that reflect the unique properties of neuromorphic hardware.
Looking ahead, the next few years are expected to see the formalization of device-level standards, particularly around interface specifications and security requirements. The European Union and other regulatory regions are anticipated to introduce guidelines for the deployment of neuromorphic systems in critical infrastructure, building on the groundwork laid by industry and standards bodies. As neuromorphic devices move from research labs to commercial deployment, adherence to robust standards will be essential for market acceptance and regulatory approval.
Investment Trends and Funding Landscape
The investment landscape for device-level neuromorphic computing systems in 2025 is characterized by a blend of strategic corporate funding, government-backed initiatives, and increasing venture capital interest. As the demand for energy-efficient, brain-inspired hardware accelerates, stakeholders are channeling resources into both established semiconductor giants and innovative startups developing next-generation neuromorphic devices.
Major semiconductor companies are at the forefront of this trend. Intel Corporation continues to invest in its Loihi neuromorphic research platform, with ongoing funding directed toward scaling up device complexity and integration with conventional AI workflows. Similarly, IBM is advancing its TrueNorth chip architecture, with recent announcements highlighting collaborative funding with academic and government partners to push device miniaturization and on-chip learning capabilities.
In Europe, Infineon Technologies AG and STMicroelectronics are leveraging public-private partnerships, particularly under the European Union’s Horizon Europe program, to accelerate the commercialization of neuromorphic hardware. These efforts are often tied to broader initiatives in edge AI and autonomous systems, reflecting the strategic importance of neuromorphic devices for future digital infrastructure.
Startups remain a dynamic force in the sector. Companies such as SynSense (formerly aiCTX), based in Switzerland and China, have secured multi-million dollar funding rounds from both corporate and institutional investors to develop ultra-low-power neuromorphic processors for edge applications. In the United States, BrainChip Holdings Ltd has attracted significant investment for its Akida neuromorphic platform, with a focus on commercial deployment in automotive and industrial IoT.
Government funding is also pivotal. The U.S. Department of Energy and the Defense Advanced Research Projects Agency (DARPA) continue to allocate grants and contracts for neuromorphic device research, targeting breakthroughs in materials, device architectures, and scalable manufacturing. These programs often involve collaborations with leading universities and industry partners, aiming to maintain technological leadership in this emerging field.
Looking ahead, the funding landscape is expected to remain robust through 2025 and beyond, driven by the convergence of AI, edge computing, and the need for energy-efficient hardware. As device-level neuromorphic systems move closer to commercial viability, investment is likely to shift from pure research toward pilot production and ecosystem development, with increased participation from both strategic investors and public sector stakeholders.
Future Outlook: Disruptive Potential and Long-Term Impact on AI Hardware
Device-level neuromorphic computing systems are poised to become a transformative force in AI hardware, with 2025 marking a critical juncture for their commercial and technological maturation. These systems, inspired by the structure and function of biological neural networks, promise to deliver orders-of-magnitude improvements in energy efficiency, latency, and parallelism compared to conventional von Neumann architectures.
In 2025, several industry leaders are expected to advance the field from research prototypes to early-stage deployment. Intel Corporation continues to develop its Loihi neuromorphic research chips, which utilize digital spiking neural networks to demonstrate low-power, event-driven computation. Intel’s roadmap suggests ongoing scaling of core counts and integration with conventional AI accelerators, targeting edge and robotics applications. Similarly, IBM is progressing with its TrueNorth architecture, focusing on ultra-low-power pattern recognition and sensory processing, with collaborative projects in healthcare and IoT.
On the materials and device front, companies such as Samsung Electronics and Taiwan Semiconductor Manufacturing Company are investing in next-generation non-volatile memory technologies—such as resistive RAM (ReRAM), phase-change memory (PCM), and ferroelectric FETs—that underpin synaptic and neuronal elements in neuromorphic chips. These device innovations are crucial for achieving the dense, analog-like connectivity and in-memory computation required for brain-inspired processing.
The outlook for the next few years includes the emergence of hybrid systems, where neuromorphic cores are integrated alongside traditional CPUs, GPUs, and AI accelerators. This heterogeneous approach is being explored by Qualcomm and Sony Group Corporation, both of which have demonstrated neuromorphic vision sensors and edge AI platforms. These efforts are expected to catalyze adoption in always-on sensing, autonomous vehicles, and low-power robotics.
Despite these advances, challenges remain in standardizing programming models, scaling device yields, and ensuring compatibility with existing AI software frameworks. Industry consortia and standards bodies, such as the IEEE, are increasingly involved in defining benchmarks and interoperability protocols for neuromorphic hardware.
By the late 2020s, device-level neuromorphic systems are projected to disrupt edge AI, enabling real-time, adaptive intelligence in power-constrained environments. Their long-term impact could extend to cloud-scale AI, where their efficiency and scalability may help overcome the energy bottlenecks of current deep learning infrastructure.
Sources & References
- IBM
- Toshiba Corporation
- IBM
- SynSense
- Qualcomm Incorporated
- SynSense
- BrainChip Holdings Ltd
- IEEE
- Infineon Technologies AG
- STMicroelectronics